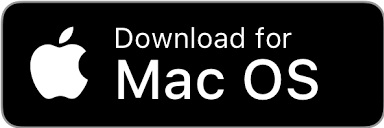
The available unsupervised classification techniques are K-Means and ISODATA. of 26Ħ Exploring Unsupervised Classification Methods Unsupervised classification can be used to cluster pixels in a dataset based on statistics only, without any user-defined training classes. Several distinct geologic and urbanization classes are also readily apparent.

Slightly darker red areas typically represent native vegetation, in this case in slightly more rugged terrain, primarily corresponding to coniferous trees. Bright red areas on the image represent high infrared reflectance, usually corresponding to healthy vegetation, either under cultivation, or along rivers. Even in a simple three-band image, it s easy to see that there are areas that have similar spectral characteristics. This image is the equivalent of a false color infrared photograph. Reviewing Image Colors The color image displayed below can be used as a guide to classification. Click Load RGB to load the image into ENVI Classic. The band names are automatically entered in the Red, Green, and Blue fields. Select Band 4, Band 3, and Band 2 sequentially from the list of bands at the top of the dialog by clicking on the band names. Red, Green, and Blue fields appear in the middle of the dialog.

Click on the RGB Color radio button in the Available Bands List. The Available Bands List appears on your screen.

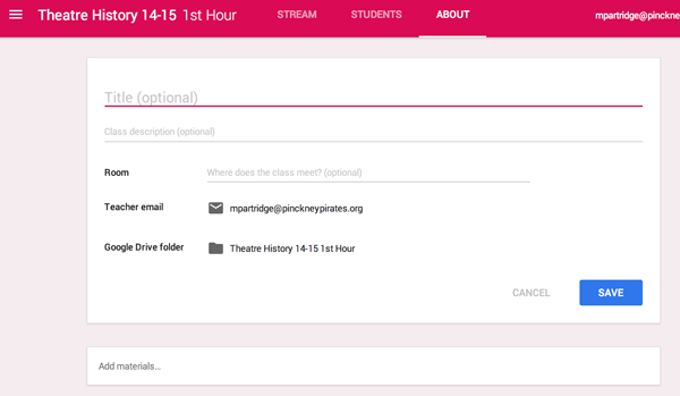
From the ENVI Classic main menu bar, select File > Open Image File. Color composite images will be used as the first step in locating and identifying unique areas for use as training sets in classification. File Description can_tmr.img Cañon City, Colorado TM reflectance image can_tmr.hdr ENVI header for above can_km.img K-means classification can_km.hdr ENVI header for above can_iso.img ISODATA classification can_iso.hdr ENVI header for above classes.roi Regions of interest (ROI) for supervised classification can_pcls.img Parallelepiped classification can_pcls.hdr ENVI header for above can_bin.img Binary encoding result can_bin.hdr ENVI header for above can_sam.img SAM classification result can_sam.hdr ENVI header for above can_rul.img Rule image for SAM classification can_rul.hdr ENVI header for above can_sv.img Sieved image can_sv.hdr ENVI header for above can_clmp.img Clump of sieved image can_clmp.hdr ENVI header for above can_comb.img Combined classes image can_comb.hdr ENVI header for above can_ovr.img Classes overlain on gray scale image can_ovr.hdr ENVI header for above can_v1.evf Vector layer generated from class #1 can_v2.evf Vector layer generated from class #2 of 26ģ Examining a Landsat TM Color Image This portion of the exercise will familiarize you with the spectral characteristics of the Landsat TM data of Cañon City, Colorado, USA.
Geogebra classic tutorial pdf download#
Files Used in this Tutorial Download data files from the Exelis website. Results of both unsupervised and supervised classifications are examined and post classification processing including clump, sieve, combine classes, and accuracy assessment are discussed. 1 ENVI Classic Tutorial: Classification Methods Classification Methods 2 Files Used in this Tutorial 2 Examining a Landsat TM Color Image 3 Reviewing Image Colors 3 Using the Cursor Location/Value 4 Examining Spectral Plots 4 Exploring Unsupervised Classification Methods 6 Applying K-Means Classification 6 Applying ISODATA Classification 7 Exploring Supervised Classification Methods 9 Selecting Training Sets Using Regions of Interest (ROI) 9 Applying Parallelepiped Classification 9 Applying Maximum Likelihood Classification 10 Applying Minimum Distance Classification 10 Applying Mahalanobis Distance Classification 11 Exploring Spectral Classification Methods 12 Collecting Endmember Spectra 12 Applying Binary Encoding Classification 13 Exploring Rule Images 15 Post Classification Processing 17 Extracting Class Statistics 17 Generating a Confusion Matrix 18 Clumping and Sieving 19 Combining Classes 20 Overlaying Classes 20 Editing Class Colors 22 Working with Interactive Classification Overlays 23 Overlaying Vector Layers 24 Converting a Classification to a Vector 24 Adding Classification Keys Using Annotation 25 of 26Ģ Classification Methods This tutorial provides an introduction to classification procedures using Landsat TM data from Cañon City, Colorado.
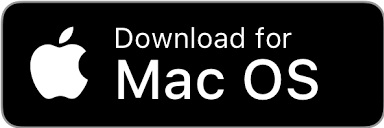